
Acooperative research and development agreement (CRADA) between the U.S. Department of Energy’s (DOE) Argonne National Laboratory and the Raytheon Technologies Research Center will allow researchers to use high-performance computing and machine-learning algorithms to reduce the computational expense of fluid dynamics models that examine coolant flow mixing and heat transfer in turbine engines, improving functionality.
“The overarching goal of this project is optimizing the combustor and turbine cooling designs for modern engines,” says Argonne research scientist Pinaki Pal, who leads the project with co-investigators Prithwish Kundu and postdoctoral appointee Opeoluwa Owoyele.
“Typically, aircraft engines operate at very high pressures with small-sized engine cores, and this tends to bring a lot of hot gases closer to the walls and increase the heat loads on combustor liner and turbine blades. This then requires a focus on thermal management,” says Michael Joly, principal investigator and research engineer at the Raytheon Technologies Research Center. “Design improvements to reduce the cooling air flow requirements can increase the engine’s thermal efficiency.”
To optimize cooling designs, researchers must consider how the cooling air is injected by selecting cooling hole angles and arrangement. Highly resolved computational fluid dynamics (CFD) simulations can achieve great accuracy but require tremendous computational expense – particularly in the boundary regions near the walls. Or researchers could use under-resolved simulations with models applied to capture near-wall flow dynamics, producing a less-accurate solution more quickly.
“The problem with the simulations that are computationally cheaper is that the wall models are not able to capture the rich flow physics prevalent in practical configurations,” Pal says.
By leveraging the power of advanced CFD modeling, high-performance computing, and deep learning, Pal and his colleagues along with industry partners from Raytheon Technologies intend to merge both approaches.
First, they’ll perform wall-resolved simulations of several engine configurations, using a massively parallel CFD code called Nek5000, recently enhanced for engine flow and combustion modeling. Researchers will use Argonne supercomputing resources, including the Theta system at the Argonne Leadership Computing Facility, a DOE Office of Science User Facility.
Then, they will take the high-fidelity data generated from these simulations to train a faster and less computationally expensive deep learning-based spatial emulator to capture near-wall heat transfer. The surrogate models will realize many of the accuracy benefits of a high-fidelity simulation, while performing computations at a fraction of its cost, according to Kundu.
Pal says, “We are creating physics-informed deep learning models that can be trained on a wide range of initial conditions to solve problems much more efficiently without sacrificing predictive capability.”
Get curated news on YOUR industry.
Enter your email to receive our newsletters.
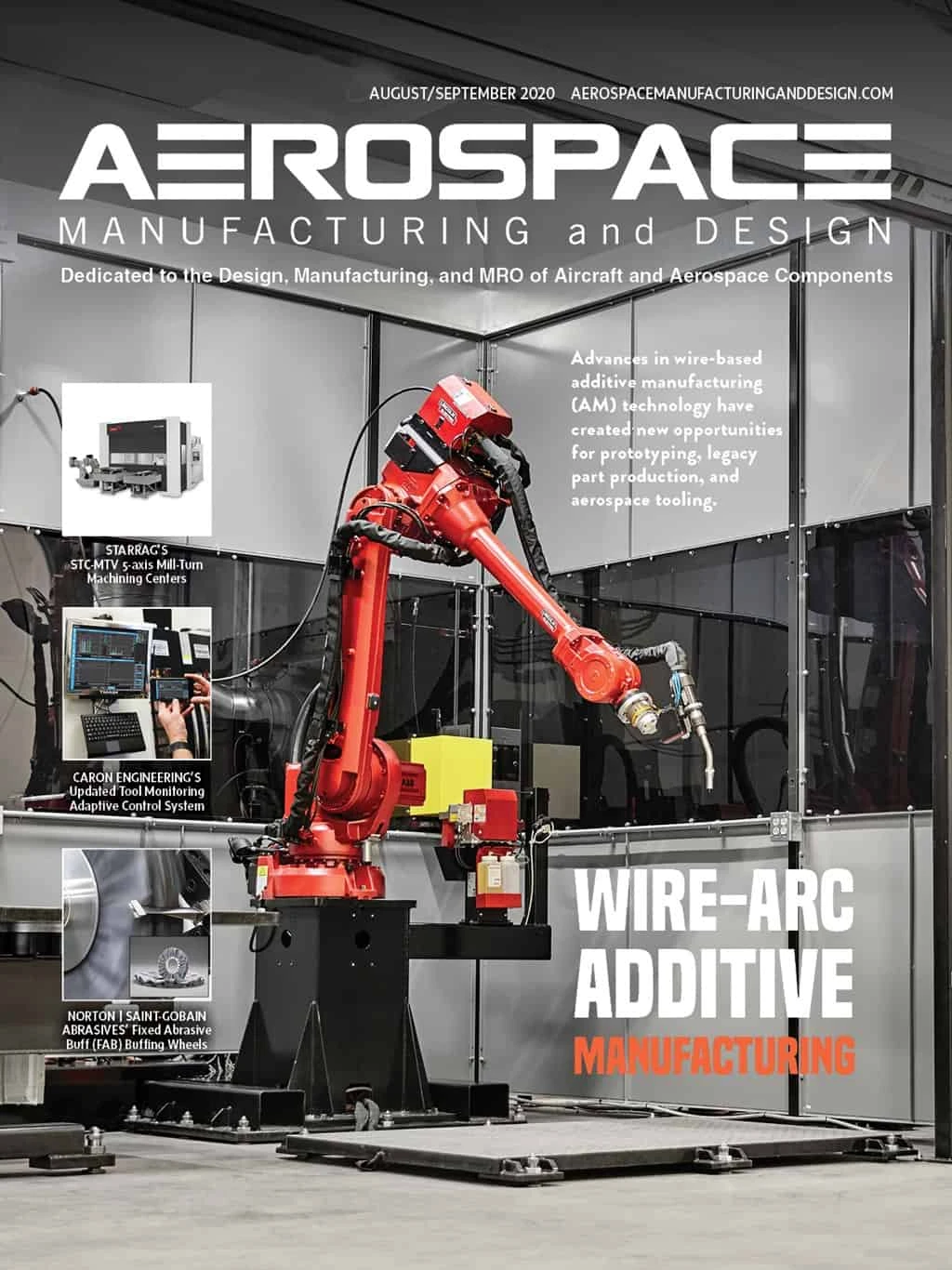
Explore the August September 2020 Issue
Check out more from this issue and find your next story to read.
Latest from Aerospace Manufacturing and Design
- Drop in for an interactive webinar on automation in manufacturing
- SW’s wing rib technology for the aerospace industry
- Mach Industries launches Mach Propulsion
- Murata Machinery USA's MT1065EX twin-spindle CNC turning center
- Electra reaches 2,200 pre-orders for its Ultra Short aircraft
- Heule's DL2 tooling for deburring of small bores
- Ralliant to establish global headquarters in North Carolina
- Philatron MIL-DTL-3432 military cables